Artificial intelligence at LifeBonus.
Bringing professional movement analysis to the care sector using artificial intelligence.
Technology for healthy movements.
People working in hospitals and care facilities perform highly demanding physical tasks often without any support to help reduce the strain on the different parts of their body. This leads to pain and long-term effects mostly in the back, shoulder and neck regions. We at LifeBonus teach people how to move better - thus leading to a more healthy body and mind. In our AI department, we build technology to make learning these movements easier.
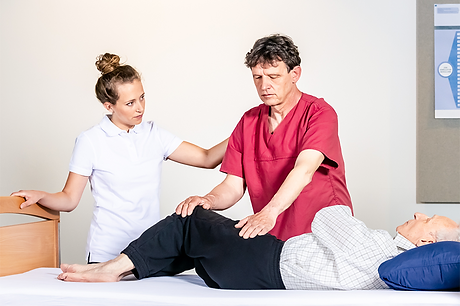

A movement analysis system to understand job-specific movements.
We are building a novel video-based movement analysis to assist the caregivers. It detects unhealthy movements from a simple video taken from a mobile phone and provides suggestions to improve those movements.
Tailoring AI to understand what’s important in a real-world care setting.
Our AI-based software learns body postures and movement patterns from videos specifically collected to understand the difficulties of people working in the care industry. We work with a combination of lab simulation and real world recordings to ensure the movement analysis solves the everyday challenges of actual caregivers.


AI with a human touch.
We build AI to work together with our human experts. Every AI output is reviewed and enriched by human specialists before reaching the end user. The human input helps to keep improving the AI. At the same time, the technology helps the human work more effectively. This gives the experts more time to focus on the really hard questions.
Recording care movements.
To analyse the ideal movement sequence, we employ sensor-based technology while capturing regular video from multiple cameras. These videos are the foundation to train our A.I. which has learnt to work without any special sensors simply by using a regular smartphone camera.
The cycle of AI project development.
An AI project is an iteration of data collection, labeling, and preparation which is followed by training a model on this data and then evaluating the results. Based on the results we make changes to each step and the process is repeated until we get the desired output. We constantly iterate to diligently improve.
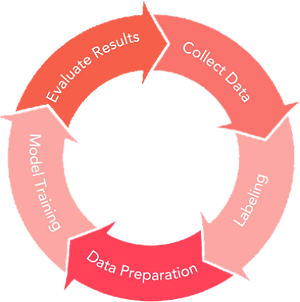